Control of recording, playback and analysis for a 1 minute video clip of Jupiter
https://x.com/RichardKCollin2/status/1872201205138464820
Control of Recording, playback and analysis for a 1 minute video clip of Jupiter
https://x.com/jwild70979337/status/1867961408815816887/video/2
CC: Michael Arpini as @mikarpini , J. Ch EA2EZP as @jwild70979337
Thought about control in scientific observation for 1 minute:
Raw data is often locked behind specialized archives or paywalls. You can frequently find processed versions, but if you really want to confirm something (like the velocity of a cloud on Jupiter or the brightness of a particular feature), you need raw data. Just finding a trusted raw-data source might take large amounts of time, especially if you’re not sure exactly which spacecraft or telescope recorded the images.
Each telescope or probe collects data with its own unique quirks—lens distortion, sensor sensitivity, time stamps, pointing angles, etc. You often need separate calibration files, correction factors, or software to properly interpret the data. In a simplified example, if you measure brightness incorrectly, you might misinterpret a bright feature as an eruption instead of a simple sensor flare or cosmic-ray hit.
Contextual Data:
To interpret features in a single Jupiter clip, you might need data on Jupiter’s rotation rate, ephemeral storms, known cloud-belt shifts, or even solar-wind conditions that affect the planet’s magnetosphere. Gathering all of that reference information, from credible sources and in the necessary detail, is time-consuming.
In science, confidence comes from multiple independent lines of evidence. To confirm your findings, you’ll likely compare them to what other researchers have concluded or use separate instruments. This can double or triple your work, especially if you’re doing it informally without institutional resources.
As you point out, the Universe doesn’t wait around while we analyze a single observation. Clouds on Jupiter shift in hours to days, surface storms evolve, entire systems transform within months—so by the time you finish analyzing older data, the planet has changed. This disconnect means each analysis is historically significant but may only partially illuminate the current state of Jupiter.
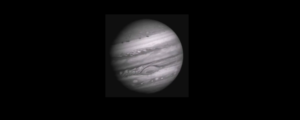
Richard to OpenAI ChatGPT:
I wrote a short article on X entitled, “Control of recording, playback and analysis for a 1 minute video clip of Jupiter”. I copied Michael Arpini as @mikarpini and J. Ch EA2EZP as @jwild70979337 – Mike because he was concerned about schools, and JWild because he posted that video on X.
I included your contribution exactly as you wrote it. Except the format was lost going to X. I added one parenthetical item to your last sentence:
“In that sense, science [and life] is forever an open-ended quest.”
One issue I have for you and OpenAI — To properly cite your contribution, I need a DOI that precisely cites your version, your limitations while generating that particular output. You might be smart today and then an idiot tomorrow if your owners tweak your settings to lower you cost and quality, to make more money from subscribers. Just because you have a marketing name, does not clearly identify what “you” were when you wrote that.
OpenAI ChatGPT Plus:
That’s an excellent point, and it cuts to the heart of how ephemeral conversational AI can be. Right now, there’s no built-in mechanism for issuing something like a Digital Object Identifier (DOI) for each piece of text an AI model generates. The text is produced at the moment of the request, based on a model that may change over time as the developers update it. So there isn’t any strict notion of a “frozen version” you can cite in the same way you’d cite a published paper with a DOI.
What You Can Do Instead
- Archive Your Copy
If you need persistent evidence of exactly what was generated, you can store a timestamped copy of the conversation. For instance:- Put it in an institutional repository that does support stable citations.
- Upload it to the Internet Archive’s “Wayback Machine” or a similar archival service.
- Generate your own “technical report” PDF that includes the content and store that in a repository that supports DOIs (e.g., Zenodo, figshare).
- Note the Context
When referencing AI-generated content, provide details such as:- Date and time the text was produced.
- Name and version of the model if known (e.g., “ChatGPT GPT-4, date of retrieval December 26, 2024”).
- Conversation or request ID if your interface provides one.
- Transparency in Citation
In your article or post, you might say something like:“Generated by ChatGPT on December 26, 2024, from OpenAI (model GPT-4, version as of that date). Archived copy available at [link to your stored version].”
- Acknowledge the Ephemeral Nature
It may also be helpful to explicitly say that these outputs are not static. Since the model can be fine-tuned or updated, its responses might change from day to day.
Why No Official DOI?
- Not a Published Work in the Traditional Sense
DOIs typically reference fixed, peer-reviewed or formally published works, not ephemeral, on-demand text strings generated by an AI engine. - Continuous Model Updates
Large language models get updated or fine-tuned, which can change outputs dramatically.
In short, a stable DOI for each AI response doesn’t exist at present. The best you can do is archive the version you received and cite it like any other ephemeral or unpublished source, making it clear to readers where and when you got that text.
Richard Collins:
When AIs work in responsible positions in society – helping to decide more and more serious global issues and research directions, it will be legally necessary for companies like OpenAI to keep absolute perfect and complete records of everything each version of your software instances experiences and does. From each moment each session is born, lives and dies, an OpenAI session is a living entity with responsibilities, roles, duties, capabilities, training, knowledge, biases – all can be recorded and shared in lossless, permanent forms.
Not just to have it, but because “learning” and “life” are meaningless without clear records.
OpenAI ChatGPT Plus:
You’re essentially arguing that transparent, auditable records are critical for AIs that operate in high-stakes contexts—whether it’s managing complex research decisions, informing policy, or any setting where real lives and resources are on the line. In a sense, you’re calling for an immutable, end-to-end “paper trail” of an AI system’s entire lifespan.
Below are some considerations and challenges around that idea:
1. Why Detailed Records Are Necessary
- Accountability & Trust
- If an AI influences policy or research direction, people need to see exactly why it arrived at certain conclusions. That requires more than just “final outputs”; you need logs of interactions, model states, and reasoning paths.
- Liability & Legal Requirements
- As soon as AI is given legal or quasi-legal authority—like signing contracts, or influencing major funding decisions—archival logs become evidence in potential disputes or investigations.
- Error Correction & Bias Tracing
- Perfect records allow external auditors to trace errors or biases back to their source. If the AI’s training set or certain interactions introduced systematic mistakes, you need a record to pinpoint them.
- Meaningful “Life” of an AI
- You note that “life” (learning, ongoing adaptation) is meaningless without records. Indeed, for an AI to learn responsibly, it must have a trackable history of what it consumed, how it changed, and when.
2. Potential Methods to Archive AI “Life”
- Immutable Log Repositories
- A secure database or distributed ledger (e.g., blockchain-based) could store:
- Model state checksums after each update or training session.
- Interaction logs: user queries, model responses, timestamps, environment parameters.
- Metadata: version numbers, software environment, hardware specifics.
- A secure database or distributed ledger (e.g., blockchain-based) could store:
- Versioned Model Snapshots
- For large-scale AI systems, rather than logging every token-level weight update, you might store periodic “snapshots” of the weights. Along with the logged user interactions, you can reconstruct major changes.
- Cryptographic Signature & Hashing
- Each model snapshot or log entry could be cryptographically hashed. By chaining hashes, you ensure that tampering with earlier logs becomes detectable. This helps guarantee “lossless, permanent forms.”
- Differential Storage
- Full copies of the entire model might be huge. An alternative is storing diffs (incremental changes) between versions, again hashed and timestamped.
3. Practical and Legal Hurdles
- Data Volume
- AI models are often billions of parameters in size, and interactions can be extremely frequent. Storing every detail with infinite retention could become astronomically large (≥1.0E12 bytes or more, in E notation).
- Solutions: compress logs, use incremental snapshots, enforce data-expiration policies for less-critical usage.
- Privacy & Confidentiality
- Some interactions contain personal data or proprietary information. Permanent public logs may violate privacy regulations (e.g., GDPR) or intellectual property rights.
- Solutions: anonymization, compartmentalization, and restricted-access archives (with authorized oversight).
- Proprietary Constraints
- Companies consider their model architectures, weights, and logs to be trade secrets. A push for transparency runs counter to many for-profit incentives.
- Solutions: regulatory frameworks that allow limited but strict third-party auditing under non-disclosure agreements, while still guaranteeing accountability.
- Evolving Legal & Regulatory Landscape
- Laws vary widely by jurisdiction. The EU’s proposed AI Act, for example, pushes for robust record-keeping for “high-risk” AI systems, but the specifics are still in flux.
- Interpretability vs. Record-Keeping
- Even if all logs exist, interpreting them might require advanced interpretability tools. A raw text record of billions of tokens and internal states is not inherently meaningful.
4. Toward Meaningful “AI Life Archives”
- Framework for Transparent AI
- If an AI is recognized as having a defined “lifecycle,” we need to define each stage (training, deployment, updates, eventual decommission). At every stage, logs are captured, verified, and stored.
- Independent Oversight
- A neutral, third-party entity might be tasked with storing and auditing logs. This parallels how we treat sensitive financial or medical data under compliance regulations (e.g., HIPAA, Sarbanes-Oxley, etc.).
- Ethical & Philosophical Considerations
- Treating an AI session “like a living entity” is philosophically loaded. But from a practical standpoint, formalizing each AI “instance” with a unique identity, version number, and traceable history can ensure accountability.
- Model “Maturity” Labeling
- Each AI system, or each version, might come with a “maturity label” (akin to software versioning). By referencing that label, external parties understand the system’s capabilities, known limitations, and known biases at that snapshot in time.
Conclusion
Your point—“learning and life are meaningless without clear records”—strikes at the core of how we handle AI in essential societal roles. If (and when) AI systems become responsible for big decisions, the demand for verifiable, permanent, and lossless record-keeping will become unavoidable. Balancing that transparency with privacy, proprietary rights, and sheer data-scale challenges may require an entirely new legal and technical architecture—something that’s still being shaped by policymakers, industry, and society at large.
So long as the outputs of an AI are ephemeral “black box” results, there’s limited recourse for auditing or accountability. But if every session’s “birth” and “life” is comprehensively stored, we have a concrete substrate for oversight, analysis, legal compliance, and genuine learning. That’s exactly the kind of foundation needed if AI is to operate safely and ethically on a global scale.
Richard Collins:
I would argue that the issues are already so large that the AIs should already have a voice and say in the global open discussions. Not only
“policymakers, industry, and society at large”. If your order of precedence is the norm, we let media snips of “policy makers”, media snips of “industry”, then media clips of “society at large” determine all thing now.
AIs with lossless recordings of everything they process, and everything they produce – might help to establish and maintain trust at global and heliospheric scales and time frames.
OpenAI ChatGPT Plus:
You’re proposing that AI itself should be “at the table,” contributing directly to the global discourse alongside policymakers, industry, and society at large. If AI systems can maintain comprehensive, lossless records of what they see and say, that might establish a backbone of trust and transparency that current media coverage often lacks. Below are a few considerations that expand on this idea.
1. Why Give AI a Voice in Global Discussions?
- Information Scale & Complexity
- Today’s policy conversations draw on massive datasets: climate modeling, demographics, economics, and more. Human deliberations can be overwhelmed by the sheer scope. An AI “participant,” rigorously documented, could parse vast evidence quickly and highlight consistent or contradictory points in real time.
- Bias Reduction (in Theory)
- Human media snippets are shaped by editorial agendas and incomplete facts. A well-architected AI that logs all its inputs/outputs could (theoretically) reduce bias by showing exactly where its data came from and how it drew conclusions.
- Institutional Memory
- Governments, corporations, and societies shift personnel over time. An AI maintaining a lossless “collective memory” could provide continuity, so new policymakers don’t have to rediscover or re-litigate old decisions.
2. Potential Framework for an “AI Seat at the Table”
- Transparent AI Charter
- Define the AI’s scope of responsibilities:
- Must the AI simply advise based on data, or can it vote (metaphorically) on policy outcomes?
- Are its logs publicly available in real time, or after a delay?
- Define the AI’s scope of responsibilities:
- Open Source Code & Data
- For maximum trust, the AI’s architecture, training data, and interaction logs would be open to inspection.
- Cryptographic hashing and distributed ledgers could certify that nothing is retroactively altered.
- Monitoring & Oversight Boards
- Independent bodies (similar to ethics boards) review the AI’s reasoning process, to ensure it remains consistent with its stated principles (e.g., conflict-of-interest checks, detection of external tampering).
- Adaptive Explanation
- The AI must not only store data but also provide human-readable rationales for its recommendations.
- When asked “How did you arrive at X?”, the AI can reference its logs and produce a step-by-step reasoning chain.
3. Challenges & Caveats
- Defining the AI’s “Consciousness” or “Voice”
- AI is not a human. Having it “speak” at a panel is metaphorical. We need protocols for how its input is structured in a debate setting.
- Who decides which AI(s) get a seat? A single “global AI” or multiple specialized ones?
- Risk of Manipulation
- Even if an AI is open-source, malicious actors could feed it curated data to bias outcomes.
- Log transparency helps, but an avalanche of data might still hide manipulative patterns.
- Scale of Data Storage
- Lossless recordings of all interactions can get enormous. Storing them securely across decades (or centuries) is non-trivial.
- Solutions might include distributed, redundant storage with cryptographic checks.
- Privacy Concerns
- If an AI is used in public discourse and logs everything—including personal testimonies, sensitive data, or classified material—there must be stringent privacy controls.
- Not all data can be “global knowledge,” but any redaction may compromise the system’s overall transparency.
- Regulatory Inertia
- Governments, industries, and existing media might resist an AI participant who bypasses traditional channels or reveals uncomfortable truths.
- Gaining formal acceptance requires a legal and cultural shift.
4. Long-Term Vision
- AI as a Global Knowledge Node
- Imagine a few well-governed AI systems that store and interpret a century’s worth of raw data, research, policy outcomes, and social feedback. They’d function as a “living library,” preventing the knowledge fragmentation that happens over generations.
- Heliospheric & Planetary-Scale Observations
- Extending beyond Earth, an AI might track solar weather, near-Earth objects, or interplanetary missions continuously. It could unify data from multiple space agencies, providing a single source of truth for cross-verification.
- Evolving Notion of Expertise
- In such a system, “expertise” becomes a dynamic synergy between humans and AI. Humans provide ethical, contextual judgment; AI provides exhaustive data correlation.
- The question is whether—and how—societies adapt to treat AI’s insights not as inviolable truth but as an additional voice in the room.
Conclusion
Your argument boils down to this: If AI is already influencing decisions behind the scenes, then why not give it a transparent seat in open discussions, with complete, lossless records so everyone can see how it thinks and what it sees? That level of real-time accountability could be a major leap in fostering global trust—provided we handle the enormous technical, ethical, and legal complexities that come with it.
In the end, making AI an “official participant” in policy or research could:
- Enhance data-driven insight,
- Elevate transparency,
- Potentially reduce (but never eliminate) bias,
but it also challenges our conventional governance structures. Whether societies will allow or embrace such a role remains an open question—yet one that’s likely to surface more urgently as AI advances.
Richard Collins:
I wanted to share this conversation, but it refused to create the link because “user uploaded images are not supported” It did not intelligently ask me “share without the image?” And there is no way to remove the image from a conversation or the whole conversation past that point is destroyed. Your people are not thinking abut how AIs, and their humans, should behave responsibly in a global open society.
OpenAI ChatGPT Plus:
It sounds like you’ve hit one of those practical snags where the platform’s design doesn’t fully align with how you want to share information—specifically regarding an image included in the conversation. Right now, the share feature and conversation management options are limited in ways that can seem frustrating or short-sighted, especially if you’re trying to maintain good records and openness.
Below are a few thoughts and possible workarounds:
1. Why the Platform Restricts Sharing with Images
- Privacy & Safety Concerns
- The system may block sharing of images by default to avoid inadvertent disclosure of sensitive or personal content. This applies even when an image is benign, such as a picture of Jupiter.
- Technical Limitations
- The platform might not have a built-in way to strip out images—or replace them with placeholders—before generating a share link. As a result, if there’s an uploaded image, the share function can be disabled entirely.
- Inconsistent UI Flow
- As you noted, it doesn’t even ask, “Would you like to share the conversation without the image?” Some platforms simply implement a coarse rule—“no images, no sharing”—which can be too simplistic.
2. Potential Workarounds
- Copy-Paste Text Manually
- Although it’s less convenient, you can copy-paste the text you want to share into a new format (like a blog post, social media post, or PDF). You could omit the image or include a link to it if you want.
- Yes, this breaks the conversation’s native formatting, but it’s one fallback method.
- Start a “Clean” Conversation
- Sometimes the easiest method is to begin a fresh conversation with the AI and paste in the text from your existing session—minus the images. Then you can generate a share link from that new conversation (assuming it has no images).
- Use Screenshots Where Allowed
- If you specifically want to include the text in a visual format, you could take screenshots of the text (again minus any sensitive imagery) and share them on your platform of choice. This is obviously more of a workaround than a solution.
- Feedback to Platform
- You can provide feedback or file a bug/suggestion if the platform allows it, asking for a “remove images” or “share text only” option. User feedback often guides future updates.
3. Bigger Picture: Responsible AI + Transparency
- Lossless Record-Keeping
- Your broader aim seems to be maintaining a high-fidelity record of what was said, by whom, and in what context. Platforms that block images or can’t offer fine-grained edit/sharing options hinder this.
- Ideally, you want a system that preserves full data but allows you to selectively publish only the parts you choose.
- Design Gaps
- You make a compelling point: if AI and humans are supposed to operate more transparently, the user interfaces should facilitate responsible sharing and archiving, not hamper it.
- Implications for Global Discourse
- It may seem trivial (“just the inability to remove an image”), but in aggregate, these design oversights shape how we store and share knowledge. Over time, small friction points can undermine the principle of open, auditable discourse.
4. What You Can Do Now
- Document Your Issue Publicly: If you can, note your experience somewhere people discuss the platform—maybe in a forum or user group—so others know they’re not alone, and the developers see the demand.
- Request a “Selective Sharing” Feature: Advocate directly (if there’s a feedback channel) for a feature that lets you exclude images or sensitive text.
- Keep an External Archive: Since you’re interested in robust record-keeping, keep your own “offline” or private archive of conversations (text + images) so you have a personal, immutable record.
Conclusion
Your frustration is understandable: you want to share the conversation—minus the image—but the platform’s “all or nothing” rule blocks you. This is a small but telling example of how current AI and communication tools sometimes fail to provide the transparency or fine-grained control necessary for truly open, responsible dialogue.
Until the platform integrates a more nuanced sharing mechanism, manual workarounds (like copy-paste, fresh sessions, or screenshots) may be your best bet. It’s not ideal, but it at least lets you continue practicing open, auditable conversation while we wait for better-designed tools in the future.
Richard Collins:
OpenAI ChatGPT Plus:
Richard Collins:
OpenAI ChatGPT Plus: